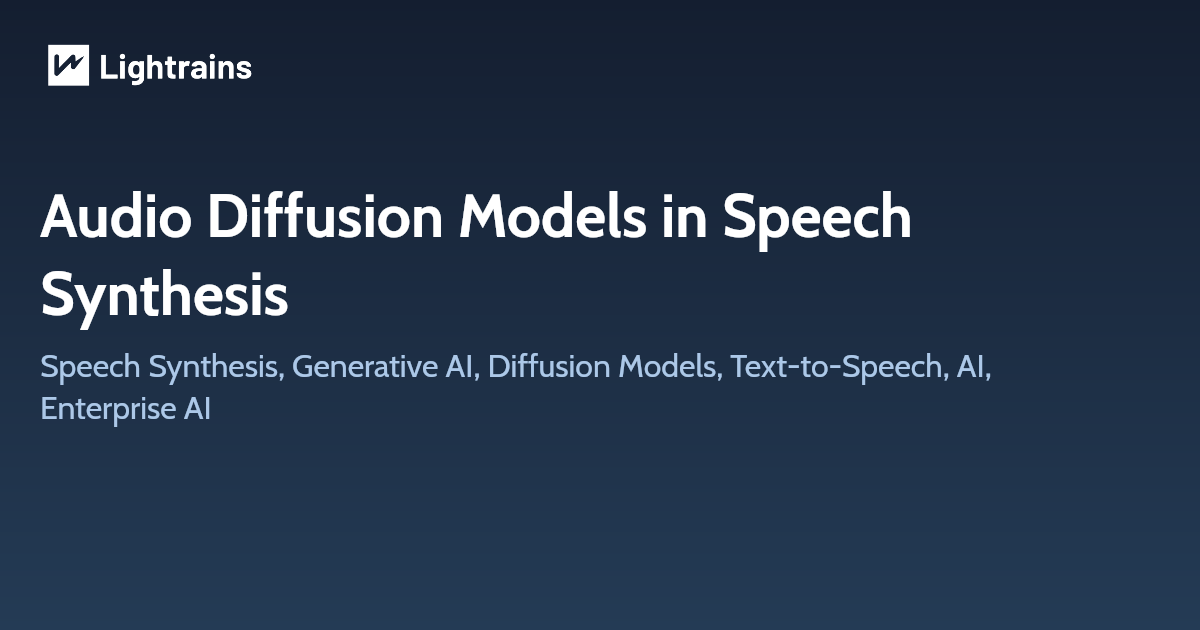
Generative AI has been making waves across various industries, and one of the most exciting areas of development is in speech synthesis. With the advent of diffusion models, the field of text-to-speech (TTS) and speech enhancement has seen remarkable progress. This blog delves into the latest advancements in audio diffusion models, offering a comprehensive overview of how these models are transforming the way we generate and enhance speech.
What Are Audio Diffusion Models?
Understanding Diffusion Models
Diffusion models are a class of generative models that have gained significant attention for their ability to generate high-quality data. The core idea behind diffusion models is to gradually add noise to data and then learn to reverse this process to generate new data. This approach has been particularly successful in computer vision, and it is now being applied to audio and speech synthesis.
The Role of Diffusion Models in Speech Synthesis
In the context of speech synthesis, diffusion models are used to generate high-quality speech from text (TTS) and to enhance the quality of existing speech (speech enhancement). These models have shown great promise in producing natural-sounding speech, making them a valuable tool in applications like virtual assistants, audiobooks, and voiceovers.
Text-to-Speech Synthesis: A Deep Dive
Evolution of Text-to-Speech Frameworks
The development of text-to-speech (TTS) systems has evolved from three-stage frameworks to more efficient two-stage frameworks. Early TTS systems relied on formant synthesis and concatenative synthesis, which were later replaced by statistical parametric speech synthesis (SPSS). With the advent of neural networks, TTS systems have become more sophisticated, leading to the current dominance of neural network-based TTS.
Diffusion Models in TTS
Recent advancements in TTS have leveraged diffusion models to generate high-quality speech. These models are typically used in two stages:
- Acoustic Model: Converts text into acoustic features (e.g., Mel-spectrogram).
- Vocoder: Converts acoustic features into waveform audio.
Acoustic Models
Pioneering Works: Early works like Diff-TTS and Grad-TTS applied diffusion models to generate Mel-spectrograms from text.
Efficient Acoustic Models: Techniques like knowledge distillation and Denoising Diffusion GANs (DiffGAN-TTS) have been introduced to speed up the generation process without compromising quality.
Adaptive Multi-Speaker Models: Models like Grad-TTS with ILVR and Grad-StyleSpeech enable zero-shot speaker adaptation, allowing the generation of speech in the voice of a target speaker without additional training.
Discrete Latent Space: Some models, like Diffsound and NoreSpeech, use discrete latent spaces to improve generation efficiency and robustness.
Fine-Grained Control: Models like EmoDiff allow for fine-grained control over emotional expression in generated speech.
Vocoders
Pioneering Works: WaveGrad and DiffWave were among the first to apply diffusion models to vocoders, bridging the gap between non-autoregressive and autoregressive methods.
Efficient Vocoders: Techniques like bilateral denoising diffusion models (BDDM) and InferGrad have been developed to reduce inference time while maintaining high-quality output.
Statistical Improvements: Models like PriorGrad and SpecGrad have introduced adaptive noise priors and spectral envelope conditioning to improve speech quality.
End-to-End Frameworks
Pioneering Works: WaveGrad 2 and CRASH have demonstrated the feasibility of end-to-end TTS systems that generate waveform audio directly from text.
Fullband Audio Generation: Models like DAG and Iton have extended the capabilities of end-to-end frameworks to generate fullband audio, offering higher quality and diversity.
Speech Enhancement: Improving Audio Quality
Enhancement by Removing Perturbations
Speech enhancement aims to improve the quality of degraded audio by removing unwanted noise or reverberation. Diffusion models have been applied to this task with great success.
Time-Frequency Domain
Pure Generative Models: SGMSE and SGMSE+ use stochastic differential equations (SDEs) to enhance speech in the time-frequency domain, achieving state-of-the-art results.
Unsupervised Restoration: UVD and Refiner leverage diffusion models for unsupervised dereverberation and speech enhancement, respectively.
Time Domain
Pioneering Works: DiffuSE and CDiffuSE apply diffusion models to denoise speech in the time domain, offering robust performance even in challenging conditions. Enhancement by Adding Missing Parts
Audio Super-Resolution
Pioneering Works: NU-Wave and NU-Wave 2 have set new benchmarks in audio super-resolution, generating high-quality 48kHz audio from lower-resolution inputs. Improved Sampling and Model Architecture: Techniques like improved sampling and modified DiffWave have further enhanced the quality of super-resolved audio.
Recent Breakthroughs (2023-2024)
AudioCraft and AudioGen
Meta’s AudioCraft framework, released in 2023, represents a significant leap in audio generation. It includes AudioGen for sound generation and MusicGen for music creation, demonstrating the versatility of diffusion models in handling various audio tasks. These models can generate high-fidelity, diverse audio samples from text descriptions.
Stable Audio
Stability AI’s Stable Audio, launched in late 2023, brings professional-grade music generation capabilities using advanced diffusion techniques. It showcases how diffusion models can be scaled for commercial applications while maintaining high quality output.
Voice Cloning Advancements
Recent developments in voice cloning technology, such as ElevenLabs’ multilingual voice cloning system, have demonstrated how diffusion models can be used to create highly realistic voice replicas while addressing ethical concerns through built-in safeguards.
Real-Time Processing
New optimization techniques like Progressive Distillation and Knowledge Distillation have significantly reduced the inference time of audio diffusion models, making real-time applications more feasible.
Future Directions
Multimodal Integration
The convergence of audio diffusion models with other modalities (text, video, images) is creating new possibilities for:
- Lip-sync generation
- Audio-visual coherence in virtual environments
- Cross-modal generation and translation
Efficiency Improvements
Ongoing research focuses on:
- Reduced computational requirements
- Faster inference times
- Lower memory footprint
- Enhanced quality-speed tradeoffs
Personalization and Control
Advanced control mechanisms are being developed for:
- Fine-grained emotion control
- Speaker style transfer
- Accent modification
- Prosody manipulation
The integration of diffusion models into speech synthesis and speech enhancement has opened up new possibilities for generating high-quality, natural-sounding audio. From text-to-speech systems that can adapt to different speakers and emotions, to speech enhancement techniques that can remove noise and restore missing parts, these models are pushing the boundaries of what’s possible in generative AI.
As the field continues to evolve, we can expect even more sophisticated applications of audio diffusion models, making them an indispensable tool in the AI-generated content (AIGC) landscape. Whether you’re a C-level executive looking to understand the latest trends in AI or a developer exploring new tools for speech synthesis, the advancements in audio diffusion models are worth keeping an eye on.
This article originally appeared on lightrains.com
Leave a comment
To make a comment, please send an e-mail using the button below. Your e-mail address won't be shared and will be deleted from our records after the comment is published. If you don't want your real name to be credited alongside your comment, please specify the name you would like to use. If you would like your name to link to a specific URL, please share that as well. Thank you.
Comment via email