Overview
In the manufacturing industry, conveyor belts serve as the backbone of material transport, efficiently carrying products through various stages of production. However, traditional conveyor belt systems often lack the sophistication to effectively monitor and analyze material flow, resulting in potential inefficiencies and production bottlenecks. To address these challenges, AI-powered computer vision solutions have emerged as transformative tools, empowering manufacturers to optimize conveyor belt operations and achieve remarkable gains in productivity, quality, and safety
Delving into the Technology
At the heart of AI-powered computer vision for conveyor belt optimization lies a sophisticated blend of cutting-edge algorithms and advanced hardware. High-resolution cameras capture real-time images of the conveyor belt, which are then fed into a powerful computer system equipped with AI algorithms. These algorithms meticulously analyze the captured images, extracting valuable insights from the visual data.
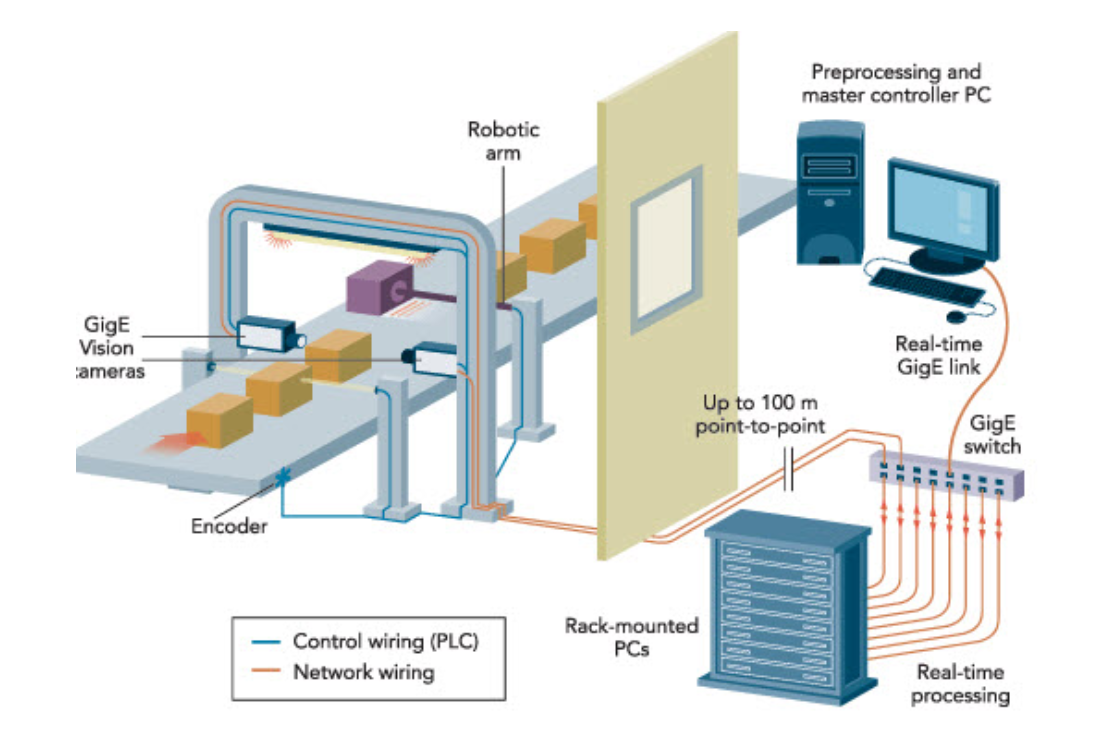
Addressing the Limitations of Traditional Conveyor Belt Monitoring.
Traditional conveyor belt systems face several limitations that hinder their effectiveness, including:
- Manual Defect Detection: Relying on human inspectors to detect product defects is prone to human error and fatigue, leading to inconsistencies and missed defects.
- Unplanned Downtime: Unforeseen equipment malfunctions or blockages can cause unexpected downtime, disrupting production schedules and increasing costs.
- Inefficient Inventory Management: Manual tracking of inventory levels can lead to discrepancies and stockouts, hindering production planning and customer fulfillment.
Proposed Solution
The implementation of CV and ML technologies can significantly enhance conveyor belt operations by addressing the aforementioned challenges:
Computer vision system and a machine learning system
The image shows a conveyor belt with a computer vision system and a machine learning system. The computer vision system is used to inspect the products on the conveyor belt for defects. The machine learning system is used to predict when the conveyor belt is likely to fail. This image illustrates how computer vision and machine learning can be used to enhance conveyor belt operations. By detecting defects and predicting failures, these technologies can help to improve safety, efficiency, and reliability.
Here is a more detailed explanation of how each technology is used:
- Computer vision: The computer vision system uses cameras to capture images of the products on the conveyor belt. These images are then analyzed by software to identify defects. For example, the software might be able to identify cracks, tears, or missing components.
- Machine learning: The machine learning system uses data from the computer vision system to predict when the conveyor belt is likely to fail. This data might include information about the age of the conveyor belt, the type of products being transported, and the operating conditions.
By combining these two technologies, organizations can create a comprehensive conveyor belt monitoring system that can help to prevent problems and improve safety and efficiency.
Here are some examples of how this system might be used:
- A food processing company could use the system to detect defects in food products, such as cracks in eggs or mold on bread. This would help to ensure that only safe and high-quality products are shipped to customers.
- A manufacturing company could use the system to predict when conveyor belts are likely to fail. This would allow them to schedule preventive maintenance and avoid unplanned downtime.
- A warehouse could use the system to optimize the flow of products through the warehouse. By knowing when conveyor belts are likely to fail, the warehouse could reroute products to other conveyor belts or take other steps to avoid disruptions.
Overall, the implementation of computer vision and machine learning technologies can significantly enhance conveyor belt operations. By improving safety, efficiency, and reliability, these technologies can help organizations to save money and improve their bottom line.
Embracing Computer Vision and AI for Proactive Conveyor Belt Monitoring
Computer vision and AI offer a groundbreaking solution to address the limitations of traditional monitoring methods. By leveraging image and video analysis techniques, computer vision and AI systems provide a comprehensive, real-time, and data-driven approach to conveyor belt monitoring.
The diagram shows a high-level overview of a computer vision system for a factory conveyor belt. The system consists of three main components:
- Cameras: Cameras are placed along the conveyor belt to capture images of the products being transported.
- Image processing unit (IPU): The IPU is a specialized computer that processes the images from the cameras to identify defects, track inventory, and predict equipment failures.
- Defect detection, predictive maintenance, and inventory tracking modules: These modules use the output of the IPU to generate real-time insights and recommendations.
The system works as follows:
- The cameras capture images of the products as they move along the conveyor belt.
- The IPU processes the images to identify defects, track inventory, and predict equipment failures.
- The defect detection module generates alerts to human operators or automated systems when defects are detected.
- The predictive maintenance module generates recommendations for scheduled maintenance tasks to prevent unexpected equipment failures.
- The inventory tracking module provides real-time updates on inventory levels, which can be used to optimize production planning.
Overall, the system helps factories to improve product quality, reduce downtime, and optimize inventory management.
Here are some specific examples of how the system can be used:
- Defect detection: The system can be used to detect defects in products such as cracks, chips, and discolorations. This can help to prevent defective products from reaching customers and improve product quality.
- Predictive maintenance: The system can be used to predict equipment failures such as bearing failures and motor burnouts. This allows factories to schedule maintenance tasks proactively, minimizing unplanned downtime and maximizing equipment uptime.
- Inventory tracking: The system can be used to track inventory levels in real time. This information can be used to optimize production planning and prevent stockouts.
The system can be customized to meet the specific needs of each factory. For example, the system can be trained to detect different types of defects in different products. The system can also be integrated with other factory systems, such as manufacturing execution systems (MES) and enterprise resource planning (ERP) systems.
Benefits
Implementing a computer vision system on conveyor belts offers a multitude of significant benefits, including:
- Improved product quality: By detecting defects early in the production process, CV systems help prevent defective products from reaching customers, enhancing brand reputation and reducing customer complaints.
- Reduced downtime: Predictive maintenance alerts generated by CV systems enable proactive scheduling of maintenance tasks, minimizing unplanned downtime and maximizing equipment uptime. This proactive approach helps prevent catastrophic failures and extends the lifespan of equipment.
- Optimized inventory management: Real-time inventory tracking provided by CV systems ensures optimal stock levels, preventing stockouts that disrupt production and excess inventory costs associated with overstocking.
- Increased productivity: Identifying and addressing production bottlenecks with the aid of CV systems helps streamline the manufacturing process, reducing production delays and increasing overall productivity.
- Enhanced safety: Monitoring equipment health and detecting potential hazards using CV systems helps prevent accidents and improve workplace safety, reducing the risk of injuries and costly liability claims.
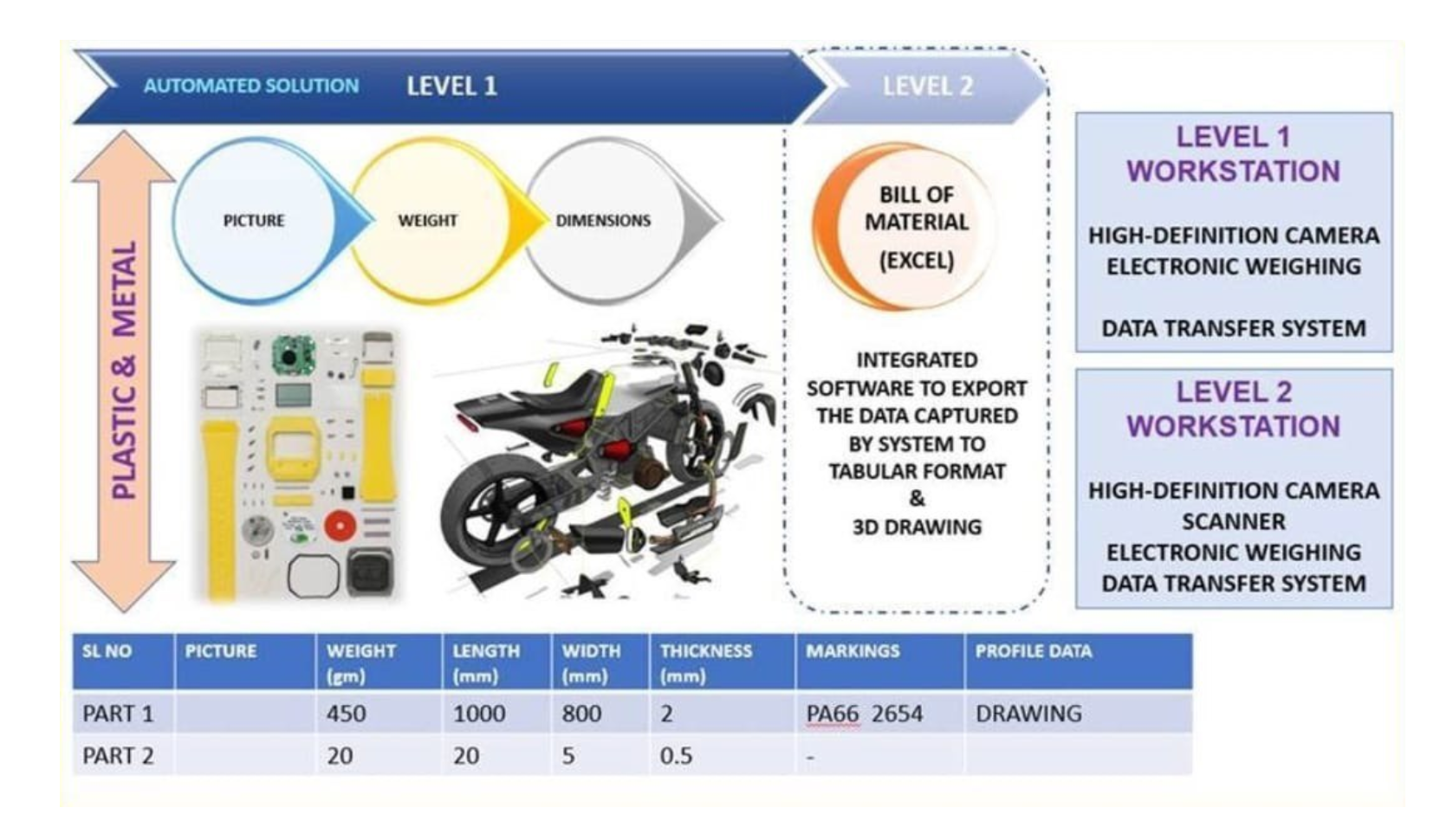
Implementation Considerations
When implementing a computer vision system on conveyor belts, several factors need to be considered:
- Camera selection: The type and placement of cameras are crucial for capturing high-quality images that can be effectively analyzed by the IPU. Factors such as resolution, lighting conditions, and camera angles need to be carefully considered.
- Image processing algorithms: The choice of image processing algorithms is critical for accurate defect detection, inventory tracking, and equipment monitoring. The algorithms need to be able to identify defects reliably, distinguish between different product types.
Additional considerations
In addition to the benefits mentioned above, CV and ML technologies offer several other advantages for conveyor belt optimization:
- Reduced Reliance on Human Labor: CV and ML technologies can automate tasks that would otherwise require human labor, such as defect detection and inventory tracking. This can free up human workers to focus on more complex and value-added tasks.
- Improved Scalability: CV and ML systems can be easily scaled to accommodate changes in production volume or product mix. This makes them a valuable tool for factories that are constantly evolving.
- Enhanced Data-Driven Decision Making: CV and ML systems can generate real-time insights into conveyor belt performance, which can be used to make better data-driven decisions.
A Practical Illustration: Transforming Operations at XYZ Manufacturing
XYZ Manufacturing, a prominent producer of consumer goods, faced chronic issues with their conveyor belt operations. Production delays, quality control concerns, and safety hazards plagued the company’s manufacturing processes. Seeking a solution, XYZ Manufacturing turned to AI-powered computer vision technology.
The implementation of the computer vision system resulted in a remarkable transformation of XYZ Manufacturing’s operations:
- Production downtime decreased by 25%
- Defect detection rates soared by 40%
- Production throughput surged by 20%
- Workplace safety incidents plummeted by 30%
- Data-driven insights empowered informed decision-making and predictive maintenance, further enhancing efficiency and reducing costs.
Conclusion
The integration of CV and ML technologies into conveyor belt systems represents a transformative step towards achieving intelligent and efficient manufacturing processes. By automating using technnologies provided by Lightrains to defect detection, predictive maintenance, and inventory tracking, these technologies empower factories to optimize production, enhance product quality, and minimize downtime. As the adoption of Industry 4.0 principles continues to accelerate, CV and ML will play a pivotal role in shaping the future of manufacturing operations.